The participative and open-access radiolarian database for automated recognition:
The open-access radiolarian images database for automated recognition using convolutional neural networks is currently composed of 24,603 images (22,559 from low latitudes and 2,044 from high latitudes), corresponding to 149 classes, of which 141 are radiolarian taxa from Neogene to recent).
Last update: 16/07/2021 (see all updates in the download section).
Keyword: Automation
The automated radiolarian recognition is part of a new automated radiolarian image acquisition, stacking, processing, segmentation, and identification workflow, described below and developed at CEREGE, in partnership with IODP-France and CNRS.
Tetard et al., published in Climate of the Past (vol 16, 2020)
“A new protocol was developed as a proposed standard methodology for preparing radiolarian microscopic slides. We mount 8 samples per slide (using 12×12 mm cover slides) on which radiolarians were randomly and uniformly decanted using a new 3D-printed decanter that minimizes the loss of material. The slides are then automatically imaged using an automated transmitted light microscope. About 500 individual radiolarian specimens (excluding the broken and overlaying specimens) are recovered from 4860 original fields of view (15 z-stacked images per FOV x 324 FOVs) per sample, after which automated image processing and segmentation is performed using a custom plugin developed for the ImageJ software. Each image is then automatically classified using a convolutional neural network (CNN) trained on a database of radiolarian images and a dedicated program”
…
“These classes were trained to be recognised with an overall accuracy of about 90 %. This whole procedure, including the image acquisition, stacking, processing, segmentation and recognition, is entirely automated and piloted by a LabVIEW interface and roughly last 1 hour per sample.”
Step 1
Microslide preparation
Radiolarian slides are prepared using a new 3D printed decanter system developed at CEREGE and composed of eight 3.5 ml tanks.
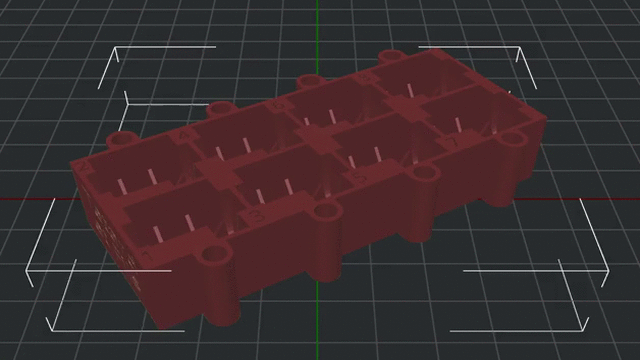
After placing a 12×12 mm cover slide in each tanks, a solution containing radiolarians in suspension is poured into each tank.
After a few minutes, radiolarians have settled on the cover slide, and water can be vacuum out using the hole on the side of each tank.
Each cover slide can then be mounted using optical glue on a glass slide (8 samples per slide) and is ready for image acquisition
3D files can be freely downloaded here.
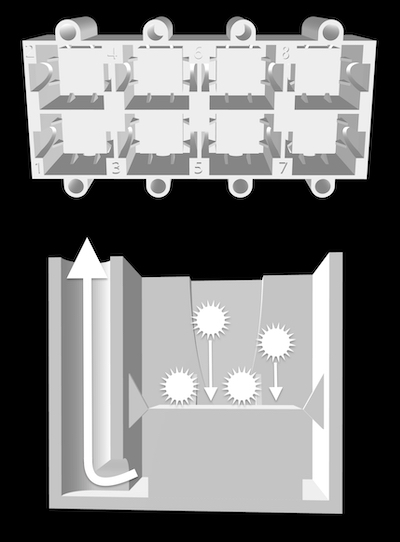
Step 2
Automated image acquisition
Radiolarian slides are automatically imaged at CEREGE using a transmitted light microscope piloted with a LabVIEW interface.
It automatically scans 18×18 fields of view (324 FOVs) on each of the 8 samples of the slide.
Each FOV is scanned 15 times at different focal depth (every 10 µm) to cover the thickness of radiolarian specimens.
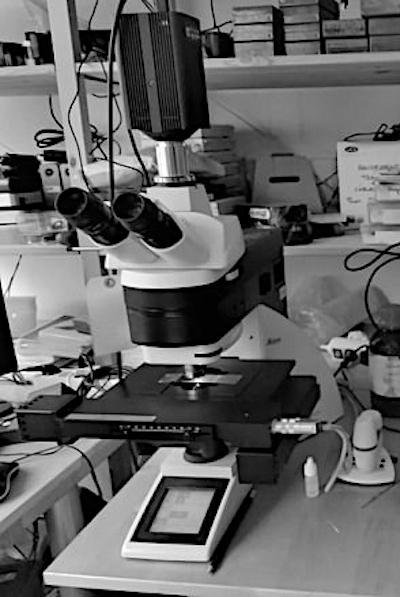
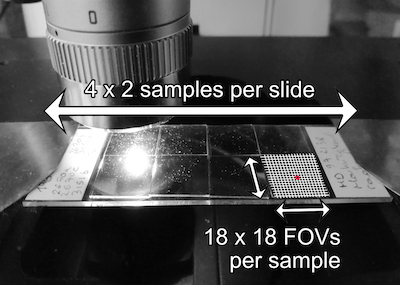
Step 3
Automated stacking
For each FOV, the batch of 15 images is automatically stacked using Helicon Focus and the depth map approach, piloted with the LabVIEW interface.
This step results in a single and clear FOV image.
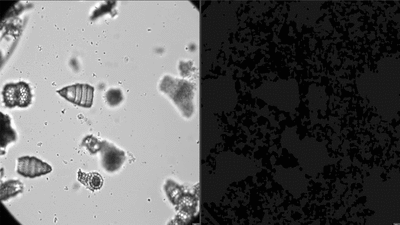
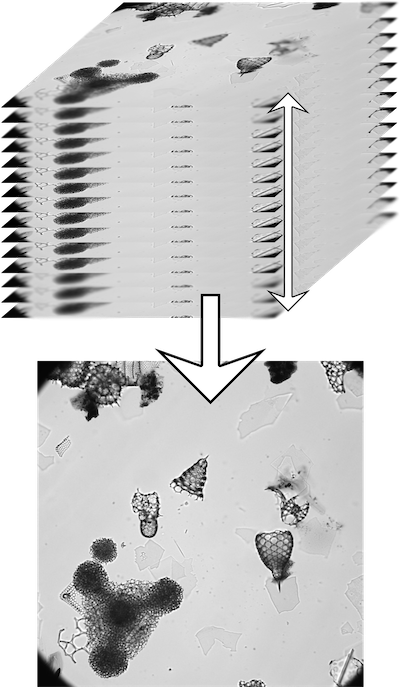
Step 4
Automated image processing and segmentation
The clean FOV image is then automatically processed using the AutoRadio_Segmenter plugin developed at CEREGE for ImageJ /Fiji and available for free here.
This plugin enhances contrast, inverts colours, subtracts background, creates Regions of Interest (ROIs) for each specimens, separates specimens in contact with each others, and saves every specimen as single individual images, for each sample.
Here is an example of segmentation on a few FOVs using the isolated plugin, slow down to see each step.
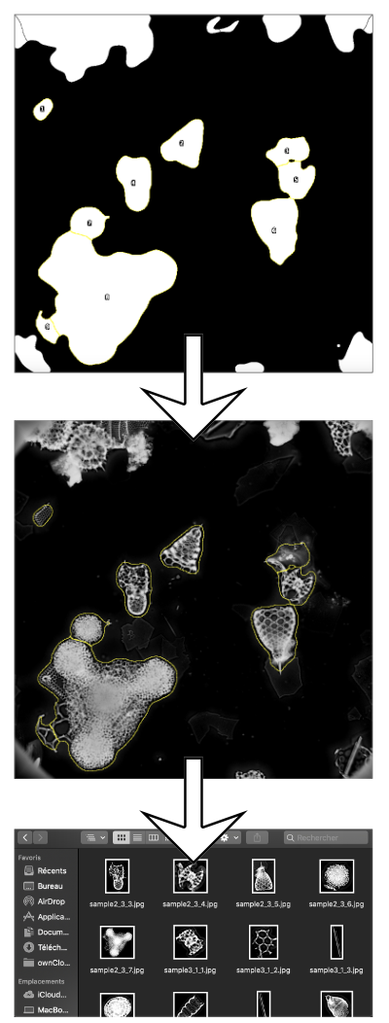
Step 5
Automated recognition
Individual images are then automatically send to a specific software developed at CEREGE (Marchant et al., 2020), that uses a trained convolutional neural network (CNN) to assign them to a class and returned their identification.
This CNN is able to recognise 124 classes of which 116 are Neogene to modern radiolarian classes with a current accuracy of 90 %. The database used to train this CNN is composed of 24,603 images, originally distributed in 149 classes, and is visible here, and can be download here.
Step 6
Automated image storage and census counts
Identified individual images are then saved into folder corresponding to their assigned classes and samples.
Census data and morphometric measurements are also automatically saved for each sample and core.
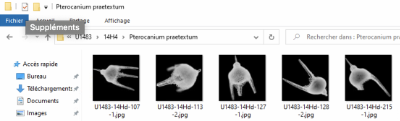
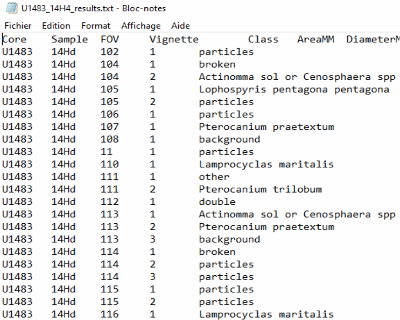
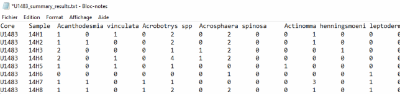
Do you wish to help with the building of the AutoRadio Database? Do you have any radiolarian images you are willing to share? Contact us!